Hyperspectral Imaging: A Non-Invasive Technique
By Lauren Grimes, graduate student, Animal Science; Govindarajan Konda Naganathan, graduate student, Biological Systems Engineering; Jeyam Subbiah, assistant professor Biological Systems Engineering, Lincoln; Chris R. Calkins, professor, Animal Science, Lincoln and published by the University Of Nebraska Extension.Summary
A hyperspectral imaging apparatus was developed and assembled to predict 14 d tenderness of beef steaks. USDA Choice and Select grade longissimus steaks (n=111) from between the 12th and 13th ribs were frozen at 14 days post-mortem, cut to 1-inch thickness, and thawed overnight for scanning, cooking, and obtaining slice shear force data. The model predicted three tenderness categories with 96.4% accuracy, correctly classifying 93 tender, nine intermediate and all five tough samples. One tender sample was misclassified as intermediate, and three intermediate samples were misclassified as tender. This hyperspectral imaging system was effective in predicting beef tenderness.Introduction
Consumers have shown a willingness to pay a premium for guaranteed tender steaks. To increase consumer satisfaction and value of beef, the industry has a strong interest in tenderness predictors. An accurate, noninvasive, online tenderness instrument is needed for packing plant scenarios. Since beef carcasses are quality and yield graded by USDA employees two days postmortem, and product typically reaches the consumer at 14 days postmortem, the machine would need to accurately predict the ultimate 14 day postmortem tenderness value.Hyperspectral imaging is a technique whereby multiple reflectance images are captured at regular intervals along a spectral axis. Thus, each pixel in a hyperspectral image has spectral reflectance data. In contrast, near-infrared (NIR) spectroscopy measures spectral reflectance of an entire field of view rather than a single pixel. Thus, hyperspectral imaging would be expected to be much more accurate as a result of the additional information that is captured.
The objective of this research project was to develop and validate an accurate, noninvasive tenderness predictor by scanning steaks at 14 days postmortem to then ultimately develop a system to predict the 14 day tenderness level (tender, intermediate, or tough) by scanning steaks at two day postmortem.
Procedure
Hyperspectral imaging apparatusA hyperspectral imaging apparatus was constructed by integrating a CCD digital video camera (Model: IPX- 2M30, Imperx Inc., Boca Raton, FL) and a spectrograph (Model: Enhanced series Imspector, Specim, Finland). The spectrograph has a spectral range of 400-1,000 nm. Spatial and spectral calibrations were performed. A diffuse-flood lighting system was designed using tungsten-halogen lamps and a dome with a white reflectance coating. Lighting was provided with six 50-W tungsten halogen lamps (Model: MR16, Phillips Lighting Co.). A lamp controller (Model: TXC300-72/120, Mercron Industries, Richardson, Tex.) converted 60 Hz AC voltage to 60 kHz. At this high frequency, tungsten halogen lamps do not respond quickly. This simulates a constant DC voltage power supply. Over the lifespan, tungsten halogen lamps get dimmer. A photodiode was placed near a tungsten halogen lamp that provides feedback to the controller. Based on the feedback, the current input to tungsten halogen lamps is increased to provide a constant intensity output. Over the lamps, a hemispherical dome of 40 cm diameter was placed, providing uniform diffuse light over the steak (Figure 1).
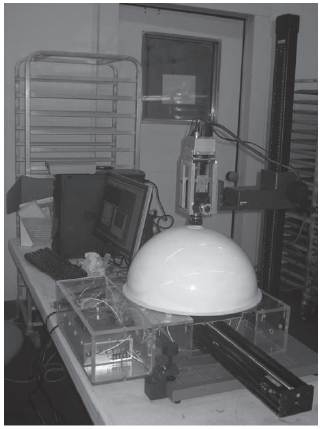
Figure 1. Hyperspectral imaging apparatus
USDA Choice and Select grade longissimus steaks from between the 12th and 13th ribs and cut to 1-inch thickness were placed on metal trays which were then vacuum packaged. The trays contained 6-14 steaks and were placed in a commercial refrigerator for a 24 hour thawing time to an internal temperature of 1-6°C. Steaks and a white reference plate were then placed on a Teflon-coated plate mounted on a linear slide that used a stepper motor for movement. The steak was then scanned by the camera to obtain a three-dimensional data cube (reflectance by twodimensional position). Scanning takes approximately 30 seconds to collect the image, and each file is approximately 600 mb. Images were obtained at wavelength intervals of 2 nm. Steaks were then cooked immediately on an impingement oven to an internal temperature of 69.5-72.2°C, and slice shear force values were obtained within one minute by an Instron Texture Analyzer.
Statistical Analysis
A 200 by 300 pixel region of the image was selected for analysis. The region of interest was in the approximate location where slice shear force samples were obtained. Principal component analysis was carried out to reduce the dimension along the spectral axis. Over 90% of the variance of all bands in the image was explained by the first five principal components. The first four principle components are shown in Figure 2. On each principal component image, co-occurrence matrix analysis was conducted to extract eight image-textural features (Figure 3); thus a total of 40 image-textural features were actually obtained from each steak. To reduce the number of features and predict 3 tenderness categories (tender - slice shear force 21 kg; intermediate 21.1 to 25.9 kg; tough 26 kg), a canonical discriminant model was developed. Leave-oneout cross validation procedures were implemented to predict the tenderness level. Figure 4 shows the hyperspectral profile of a lean vs. fat pixel.
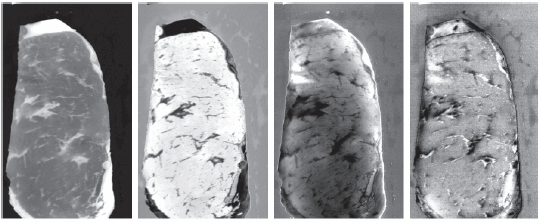
Figure 2. First four principal component images (PC # 1,2,3 & 4 from left to right).
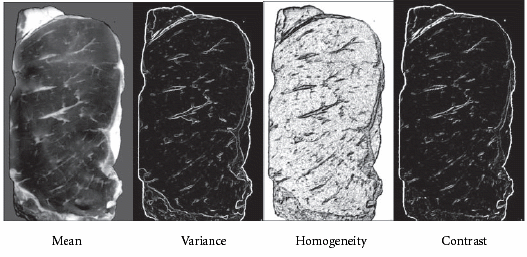
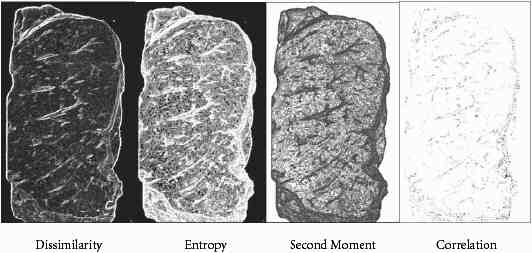
Figure 3. Co-occurrence matrix analysis to extract 8 textural features of brrf steak
Results
The model correctly classified 9 tender, nine intermediate, and five tough samples, incorrectly classi- fied three intermediate samples as tender, and incorrectly classified one tender sample as intermediate. All tough samples were correctly identi- fied. Tenderness was predicted by this hyperspectral imaging device with 96.4% accuracy (Table 1).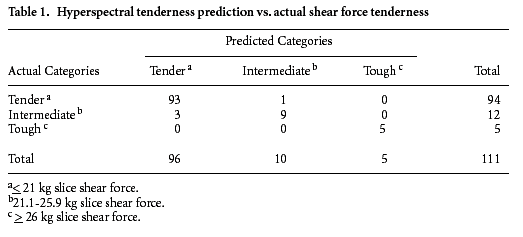
Implications
This hyperspectral imaging system was effective in accurately predicting 14 day tenderness of beef longissimus steaks. With implementation of a non-invasive, accurate tenderness predictor, beef cuts could be labeled and sold at a premium as guaranteed tender. With this premium, producers, feedlots and packing plants would reap the benefits together.Jan 2007